Attribution 2.0
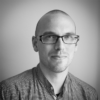
Pierre Rudolfsson
Lead Data Scientist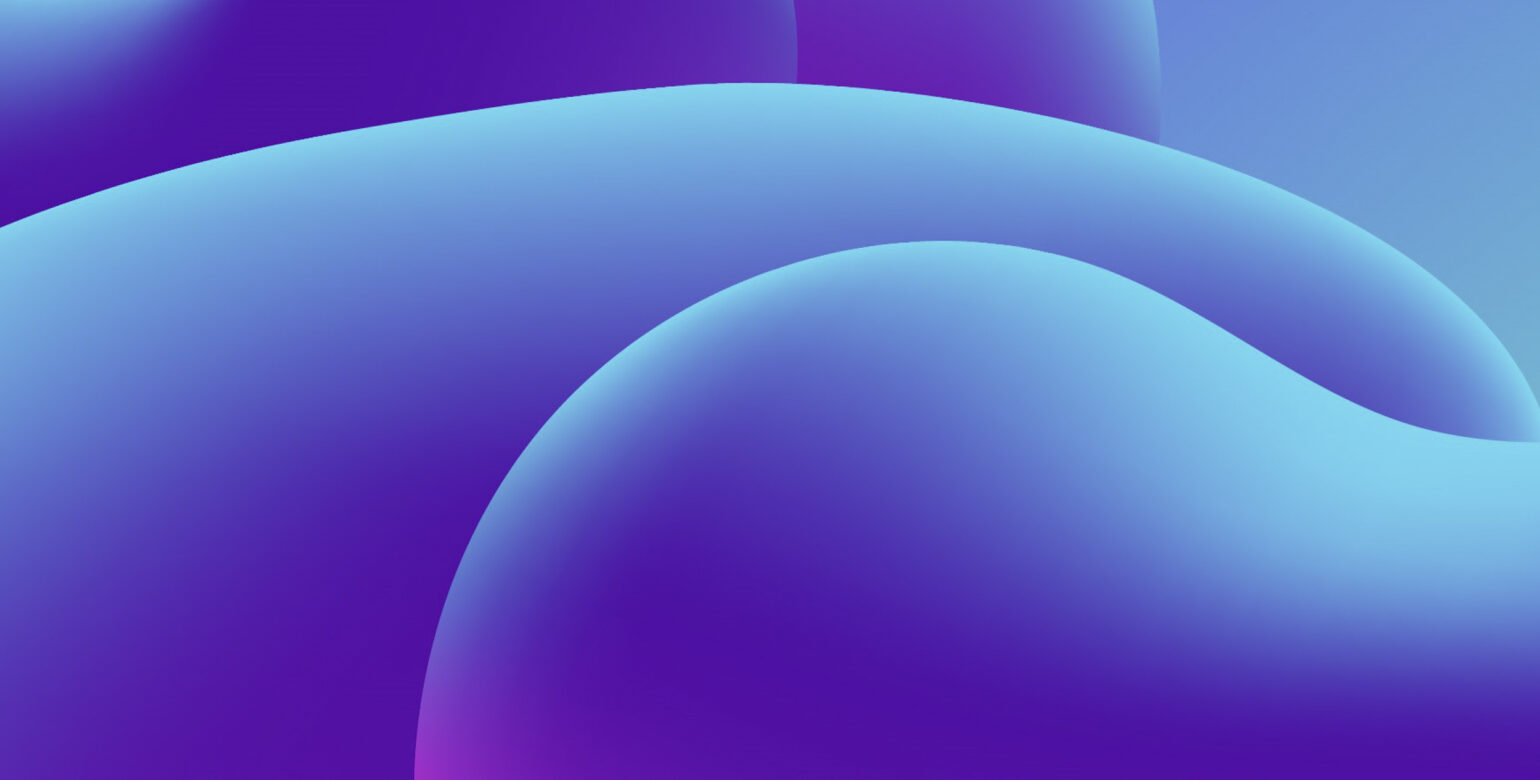
This is the last blog post in our series around “Why your main goal should NOT be to build a unified customer journey”. Throughout this series, we’ve been taking you on a customer journey from the topic of building a modern customer/marketing data warehouse to feeding ad platform algorithms. In this concluding part, we’ll get to the core of marketing, covering what will be needed for the subject of attribution.
In general, attribution is about understanding what value that marketing is generating. Currently, there are three different types of methods available. All of them have benefits and drawbacks. Let’s cover them briefly.
Path-based attribution
Most web analytics software, such as Google Analytics, measure each visit to a website or app. Together with each visit, the source is measured, and multiple sequential visits from the same unique identifier are called interaction paths.
This is done through the use of cookies, where the web analytics software is storing a cookie together with a unique identifier in the user’s browser. If you accepted cookies when entering this blog post, Google Analytics has stored a cookie in your browser with a so-called client-ID as the unique identifier. Depending on where you came from when finding this blog post, that will also be stored.
In the case of Google Analytics, client-ID is unique for each browser, meaning you’ll get a new client-ID if you visit this post through a different device or browser. This limitation is an example of a drawback we will look at later, but there is a possibility to solve for that through additional tracking (implementation of user-ID).
In its simplest form, path-based attribution is done through analysis of all user or browser interactions that took place before a transaction or conversion and then trying to figure out which interaction should receive what credit for making that happen.
Generally, there are two different types of methods: rule-based and algorithmic. An example of a rule-based model is the commonly used Last Non-Direct Click model (LND) in Google Analytics. In essence, the LND model allocates 100% of the value to the last known interaction before the event. In comparison, an algorithmic approach often referred to as data-driven, uses mathematics and statistics to decide what interaction should get what credit.
All path-based attribution models bring the assumption that the analysed interactions have an effect in the first place, which might not always hold true. Therefore, path-based attribution modelling can never answer the question of how much of the transactions or conversions would have happened anyway. That drawback leads us to the second category of attribution.
Incrementality attribution
The purpose of the incrementality attribution is to figure out how much more (incremental) effect one got from running a specific marketing activity. It is designed to answer the question that path-based attribution can not, and in addition that can be very powerful. The method is doing this by exposing one group for the ad and one not. After that, the effect is measured and compared to these two groups. The technical process for performing incrementality attribution is either based on an advertiser’s first-party data or third-party data provided by the ad platforms.
A benefit of the first-party data method is that you can control the whole methodology, from experiment design to analysis. A drawback is that it will be limited to users that have previously interacted with your website or app. It also becomes very complex, if not impossible, to analyse cross-channel effects through application of incrementality methodology.
Marketing Mix Modeling
The last category of attribution is often called Marketing Mix Modeling (MMM). The method is a quantitative regression analysis that aims to explain the effects on value based on different factors. In general, it is done by modelling a dependent target variable (e.g. revenue) against a set of independent variables (e.g. media spend). The variance in the independent variables should explain the variance in the dependent variable; if done correctly, it can be very powerful. Compared to path-based attribution and incrementality attribution it can answer the question around diminishing returns, and hence also budget optimisation – all without the use of cookies.
What does marketing attribution have to do with the overall theme around “Why your main goal should NOT be to build a unified customer journey?”. So far, the purpose of building a unified customer journey has not only been about improving the user experience; it has been as much about enhancing the optimisation of marketing investments. When it comes to optimisation of media buying, attribution is critical, and therefore related to changes in data availability.
Of these three methods, the most commonly used is path-based attribution. It is adopted widely across the whole industry of digital media buying as the core method for attribution. As with any model, the output is only as good as the underlying data, and path-based attribution relies on data. That means that using path-based attribution as a method has become worse. It will be able to identify fewer channels in their interactions, and interaction paths will be broken. This used to be a problem due to the user-ID/client-ID problem mentioned above, but with changes in privacy, it’s even more significant. If not dealt with, it will lead to relative sub-optimisation of all marketing investments, which is far from ideal.
Next-generation attribution
If you’ve found yourself stuck with a rule-based attribution model due to data-driven attribution modelling being too complicated, unfortunately, things won’t become more comfortable. With changes in data quality and the increasing demand for improvements in marketing attribution, the next generation of attribution calls for even more complexity. Path-based attribution modelling will be based on less data. Paths will be broken and channels that are identified will decrease. The next-generation attribution will have to solve this by combining the different methods explained briefly at the beginning of this blog post.
Regression analysis is not using cookie data as input, so it is a good place to start. However, a lot of innovation is needed when it comes to the concept of MMM. The traditional MMM formulation aims to explain the spend-return relations of the individual channels, but was developed in a time where channels were mainly:
- Push channels: Such as TV, radio, print, catalogues. Compared to digital marketing channels, they had a limited possibility of relevant audience targets.
- Mostly untracked: Certain actions could not be associated with a channel as a source.
- Fixed costs / few in-channel parameters: Ad cost was mostly not determined by actions on the part of the consumers, and advertisement in a channel could be viewed as buying more or less of a single product.
- This situation lends itself to regression modelling for three reasons: First, there is no data on what happens between the investment in a given channel and the measured total value, so the only way to infer causal impact is (basically) the correlation between the spend and total return.
- Second, the fixed cost and push nature of channels implies that they behave in a similar way, in particular how the spend interacts with other variables, such as season or variations in demand.
- Third, the lack of in-channel parameters to control means that the spend and return has a one-to-one relationship. We simply buy more or less of the same thing.
It’s safe to say that channels have changed. These three aspects are essential to think about since changes in these also change both the available data for MMM as well as the expected relationship between spend and return. A clear example of the importance in this is to think about the spend-return relationship for linear TV compared to Google Ads (Search):
Linear TV: Spend-return relation will be scaled by the return axis by variations in demand, meaning that the ROAS will be lower during periods of low demand, and vice versa. The cost can not be optimised at the time of the purchase. Relatively few in-channel parameters to control, making spend more or less proportional to reach / exposure.
Google Ads (Search): Both the spend and return axes will be scaled by variations in demand. Spend is not only decided by your bid, but is also affected by the number of queries. Tracking implies we know more about the causal links between the input of spend and the output of revenue.
In other words, modelling Google Ads (Search) in the same way as linear TV is inadequate: in the best case the model will be weak due to discarding relevant (tracked) information. In the worst it will yield the wrong answer due to different behaviours under varying demand or in-channel optimality.
The next-generation attribution should be a combination of path-based attribution and MMM, built on a more modern MMM approach, that is utilising the improved data at hand as well as adjusting for known differences in channel characteristics. Through that approach, attribution will once again be able to answer questions such as:
- What do we get if we spend X in channel Y?
- How much more can we spend in channel Y?
- What is the effect of channel Y if we spend X in channel Z?
Now we reach the end of our five-part series
To wrap up, the companies who create the best possible data infrastructure and evaluation will form a competitive advantage. Attribution is likely to be the foundation of all your media and user optimisation, and therefore it will affect profit. Any incremental improvement of the model, or the underlying data, will unlock high potential for improved profitability, growth or profit maximisation. Having suboptimal attribution will have the same negative effect as setting the wrong target.
As complex as it may sound, the solution is in the combination of path-based attribution modelling and MMM, where MMM is stepping in to surface missing data, and where incrementality attribution is the icing on the cake to improve the final output.
If you missed any of our other articles in the series you can catch up below:
Part 1: Why you should not build a unified customer journey
Part 2: Building a modern data warehouse
Part 3: Setting a best in class privacy strategy
Part 4: Capture, store, and activate the right data